Discussing The Algorithms That Direct Online Face Verification Systems
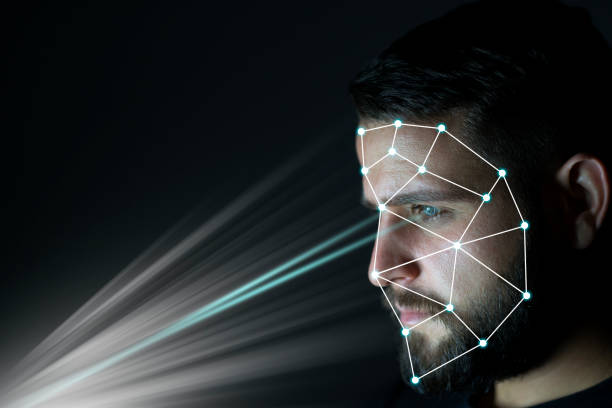
Modern facial recognition technology is shattering all uncertainties that people had in their minds about the digital matching of faces. From being able to guess ages to understanding facial expressions and emotions, online face verification technology has come a long way.
Modern online face verification solutions have a lot of applications and each one of these applications requires an extensive amount of research and industry experimentation for the technology to become more common in use.
How online face verification works – the Process
Digital face verification is an extensive process and involves several complex systems. However, from start to finish, the whole process can be divided into four phases.
Phase 1 – Detection
Once the process is signaled to be started i.e., a camera is turned on or a picture is submitted, the detection programs within the online face verification system start to look for the face. When the face, or all the faces, in the picture or screen, have been detected, the software starts to acquire the facial data of each.
Phase 2 – Pre-processing
In the second step of online facial biometrics, every face’s facial landmarks such as eyes, lips, nose, etc. are detected and facial data is acquired.
Irrelevant information such as hair on the face is removed, facial clarity is improved, and in the case of a video, all frames are combined to produce one model. Facial landmarks may be aligned, if required, in situations such as when facial perspectives are distorted due to camera errors.
To reduce noise and analyze better, the facial area gets cropped from the rest of the picture.
Phase 3 – Feature Extraction and Analysis
The third phase of the face recognition process is the most complex one. Here, complex technology such as machine learning, deep learning, etc. may be applied for automation of feature extraction for analysis of each of the facial landmarks.
The data acquired is then used to create a model called a faceprint which is unique for every individual. This faceprint may then be stored or processed through a comparator for comparison purposes.
Phase 4 – Comparison or Recognition
Comparator networks are used to compare two sets of information. In the recognition step of online face verification, the data from the face just scanned may be compared to facial data of a reference image. For example, for client face verification, the client might have provided a picture or a video sample during their digital onboarding. During the face recognition process, the facial data just submitted, let’s say, for transaction approval, will be compared against the original sample. The result will either be positive or negative for the match.
How Deep Learning Works for Face Recognition Technology
Use of deep learning facial recognition is a relatively newer integration. Within deep learning, a type of neural network called convolutional neural network or CNN is used for face recognition. The system uses layers of specificity and moves from general and broader aspects of the face such as face shape and then goes down getting more specific with each layer.
The best thing about online face verification services using deep learning models is the fact that deep learning gives the computer the ability to learn and utilize the facial features’ recognition process of the human brain. This is a step further from machine learning which gives the computer the ability to simply adapt and grow but still requires some human involvement.
Creation of 3D models for facial verification
Online face verification companies make use of 3D mapping software to create facial models using 3D facial reconstruction technology. Face verification does not require only 3D data through videos, and the data can be acquired using simple selfies as well. 2D systems for face matching are however relatively less reliable.
A 3D mapping software may measure distances between facial landmarks, geometric characters, etc. to create complex mesh structures for modeling.
Confidence Scores and what they mean for online face verification services
When dealing with a digital or online face verification service that is handling many faces and matching them across a database with even more data sets, confidence scores matter a lot. Confidence scores are numerical values that determine how alike any two datasets that are being compared are.
The lower the confidence score, the lower will be the accuracy of the faces being matched, and similarly higher confidence scores reflect higher accuracy of results when two faces are being compared.
The accuracy of an online face verification process can be impacted by a lot of factors. These factors include skin colour, lights on the subject, photo angles, etc. The existence of these factors demands an increase in efficiency and accuracy, and confidence scores promise just that by essentially setting up a feedback loop for learning.
The good thing is that businesses utilizing most online face verification services can set up confidence thresholds if thresholds are not fixed/mandated by the governing authorities. Setting a higher threshold value will decrease the chances of a mismatch, while a lower value would do the opposite.
Industry standards are important to be maintained, as so are effective security measures. These are not just essential for business owners but can help the public at large as well. With current technological trends, online face verification systems can be expected to grow and evolve much more, ultimately leading to even more effective identity verification processes in the future.
Comments are closed.